Introduction
As the Artificial Intelligence (AI) ecosystem evolves day in and day out, detection systems become very important in ensuring integrity and authenticity of content. In this drive, AI detection tools such as HireQuotient AI Detector are imperative. This paper presents a comprehensive and all-rounded piece on methods that individuals might employ in a bid to bypass these detection tools and proposes counter-strategies to attain robust AI content detection.
Understanding AI Detection Mechanism
One has to know how AI detection mechanisms work to effectively avoid them. Most of those mechanisms are based on the following elements:
Linguistic analysis: the mechanism analyzes texts for repetition, redundancy, and consistent style – a sure sign of AI generation;
Statistical patterns: unnatural frequency distributions of words and phrases are identified.
Machine learning models are trained on large volumes of data containing known AI-generated and human-written text.
Text comparison: the submitted text is checked against large databases of known AI-generated content.
Advanced AI detectors like HireQuotient follow these methods to give highly accurate and reliable content analysis.
Common Strategies to Avoid AI Detection
Paraphrasing AI-Generated Text
Through paraphrasing, sentences would be rephrased, words would change, and the structure of the text would be different to appear originally written. However, advanced AI detectors are based on semantic analysis to unveil the inner meaning. Hence the paraphrased content is detectable.
Mixing AI-Generated and Human-Written Content
The tactic in combining AI-generated content and human-written text is to dilute AI traces. AI detectors counter by using consistency checks in a context, coherence, flow to identify the mixture of sources of content.
Use of Synonym Replacement
Simple replacement of words with their synonyms is a technique. Advanced detectors check the contextual vocabulary to ensure that synonym replacement does not disrupt the natural flow and meaning of the text.
Breaking Text into Smaller Segments
This method can be rendered useless if the text is divided into small segments since most AI detection systems look for patterns in bulk text. Segment analysis, reconstruction of the same, and checking at the aggregate level of content enable AI detectors to pick out AI trends in the material.
Introduce Random Noise
Random character additions or changes in punctuation confuse detection algorithms. Cleaning algorithms filtering out noise in text reveal underlying patterns defeating this strategy.
Mobilize Multiple AI Tools
Content generated through various AI tools differs in output, thus potentially evading detection. Comprehensive pattern analysis compares text across different AI models to flag multiple tool-generated content.
Best Practices to Ensure AI Detection Accuracy
Continuous Model Training
AI detection models should be updated and trained on new datasets regularly to stay ahead of the ever-evolving techniques applied in AI generation. This also includes the diversification of data sources with frequent updates of algorithms.
Combine Multiple Detection Methods
Clearly, through the combination of various methods, including linguistic and semantic analysis, statistical and contextual analysis, guaranteed is robust detection.
Introduce Independent Auditing Mechanisms
A process of manual review of flagged content would prevent false positives and ensure that AI content has been accurately identified. It is, therefore, important to have a human-in-the-loop system with defined processes for verification.
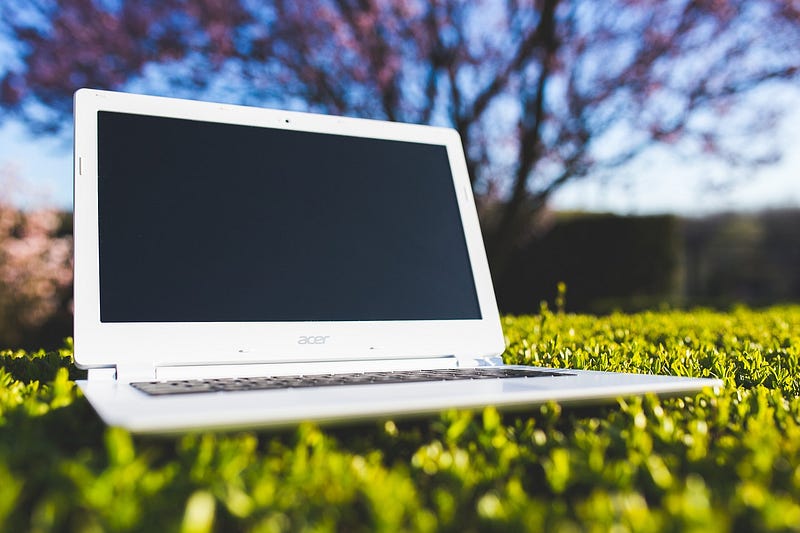
More Transparency and Education
Educating users on the authenticity of content and the AI detection tools will reduce the number of attempts at evasion. Clear communication and education regarding the preservation of integrity of content and ethical usage of AI has to be clearly conveyed.
How HireQuotient AI Detector Foils Evasion Strategies
Paraphrasing Detection
Through semantic analysis, the HireQuotient AI detector can detect paraphrased content by reading the meaning contained in the text.
Contextual Consistency Analysis
This checks for the coherence and flow of the document, looking for inconsistencies that may indicate mixed sources.
Contextual Vocabulary Analysis
This tool examines the context in which words have been used. In doing so, it makes sure that the replacement of words with synonyms does not affect the original flow of the text.
Segment Analysis and Reconstruction
The detector identifies AI-generated patterns by analyzing text segments separately and reconstructing them.
Noise Filtering Techniques
Sophisticated noise filtering techniques clean the text to bring out underlying patterns, effectively neutralizing random noise insertion strategies.
Comprehensive Pattern Analysis
Comparing the text across different AI models, it flags content from multiple tools. This would, therefore, result in full detection.
Conclusion
This makes the demand for robust AI detection tools imminent. Of course, several methods have been designed to sidetrack these tools from detection, but more advanced tools, such as the HireQuotient AI detector, counter these tactics well. Only by knowing how to detect it and putting in place best practices against content manipulation can educators, businesses, and creators be assured of content integrity and originality. This makes the HireQuotient AI detector a quite important tool in the current digital landscape toward ensuring content authenticity and reliability.
External Sources for Added Credibility:
- OpenAI’s Research on AI Text Generation
- Google’s AI and Machine Learning Research
- Stanford University’s AI and Machine Learning Resources
- MIT’s AI and Machine Learning Projects
- Harvard’s AI and Machine Learning Research